Press, PR & Media
From Tangible Interfaces to Creative AI
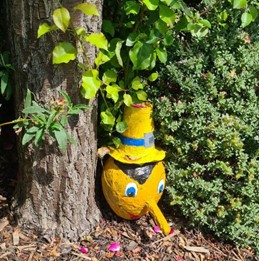
My Research Journey of AI, Wellbeing and Robotics, by Kieran Woodward
I started my PhD in 2018 at Nottingham Trent University where, I developed “Tangible Fidgeting Interfaces” – physical objects that people can manipulate while embedded sensors capture physiological data like heart rate and motion patterns. These devices provide a natural interaction method while simultaneously monitoring mental wellbeing indicators. Co-design was a fundamental aspect of this research as we worked with participants who had intellectual disabilities in order to promote the communication of emotional wellbeing. We conducted workshops and focus groups where potential users explored designs, functionality, and sensor configurations through storyboarding, real-time 3D printing, and hands-on electronics exploration. This collaborative process transformed the technical concepts into practical, user-focused solutions that people genuinely wanted to use.
One of the key advances developed during my PhD was personalised AI models by adapting a global wellbeing AI model with an individual’s small real-world dataset. By tailoring the AI models to individual users, we achieved high accuracy in wellbeing state classification – over 90% using the personalised approach. Furthermore, when just using motion data without physiological sensors, we achieved an average of 88% accuracy, which demonstrates the potential to detect wellbeing from the way people interact with the tangible interfaces. This personalisation approach has shown great benefits due to us all expressing stress and anxiety differently, so one-size-fits-all models inevitably miss important personal patterns.
Post-PhD, I applied my knowledge to “Tag in the Park,” a gamified approach to increasing physical activity. This mobile application created a location-based treasure hunt experience combining Bluetooth proximity detection, AI object recognition, and interactive educational content. The app was deployed at Rufford Abbey Country Park and utilised the features around the park to create an interactive experience; in particular when users approached sculptures in the park, the app triggered the camera where on-device AI then scanned to check whether they had found the correct sculpture, making physical activity engaging and purposeful.
We later adapted this concept for Highbury Hospital with “Tag 4 Active Lives,” creating a one-mile activity loop around the hospital grounds to promote physical activity for patients with co-designed objects for AI object recognition activity. Overall, the project successfully encouraged patients and staff to be more physically active, demonstrating how interactive technology can support wellbeing and be used to promote physical activity in an engaging and creative manner.
More recently my research has focused on edge computing, which is running AI models locally on small devices such as microcontrollers. A significant technical challenge in my work has been running sophisticated AI classification models on these resource-constrained devices. I recently implemented dual deep learning models on microcontrollers with just 256KB RAM and 1MB flash storage. This approach enabled the detection of stress using physiological sensors on-device in real-time, while reducing the impact of motion artefacts, by having a second classification model that limited stress detection during physical activity – as the physiological response of both stress and exercise is very similar. Going further, I developed a parallel processing method for dual-core microcontrollers that combined simultaneous audio and image AI classification to reduce processing time and improve accuracy by 12.27% compared to uni-modal approaches. Furthermore, my improved approach to adaptive knowledge distillation – where small “student” models learn from larger “teacher” models – achieved 86.76% classification accuracy with a 42x reduction in parameters for ultrasound plane classification. This model compression makes it feasible to deploy AI capabilities on small resource-constrained devices like wearables.
Currently, I’m exploring creative applications of AI with the Turing AI Fellowship ‘Somabotics: Creatively Embodying AI’. As part of the Embrace Angels project, I have started developing a generative AI transformer model that creates robotic movements for embracing between humans and robots. So far, we have collected a limited dataset of 9 complete “embrace” samples during a data recording session, and the AI model has been trained on this data. The trained model then enables us to generate new unique, fluid robot movements based on the previously collected data. Looking forward, I am excited about several directions including more creative AI models, a new approach to make robot movements more human-like and continued advancements in edge computing that enable increasingly sophisticated AI to run locally on devices.